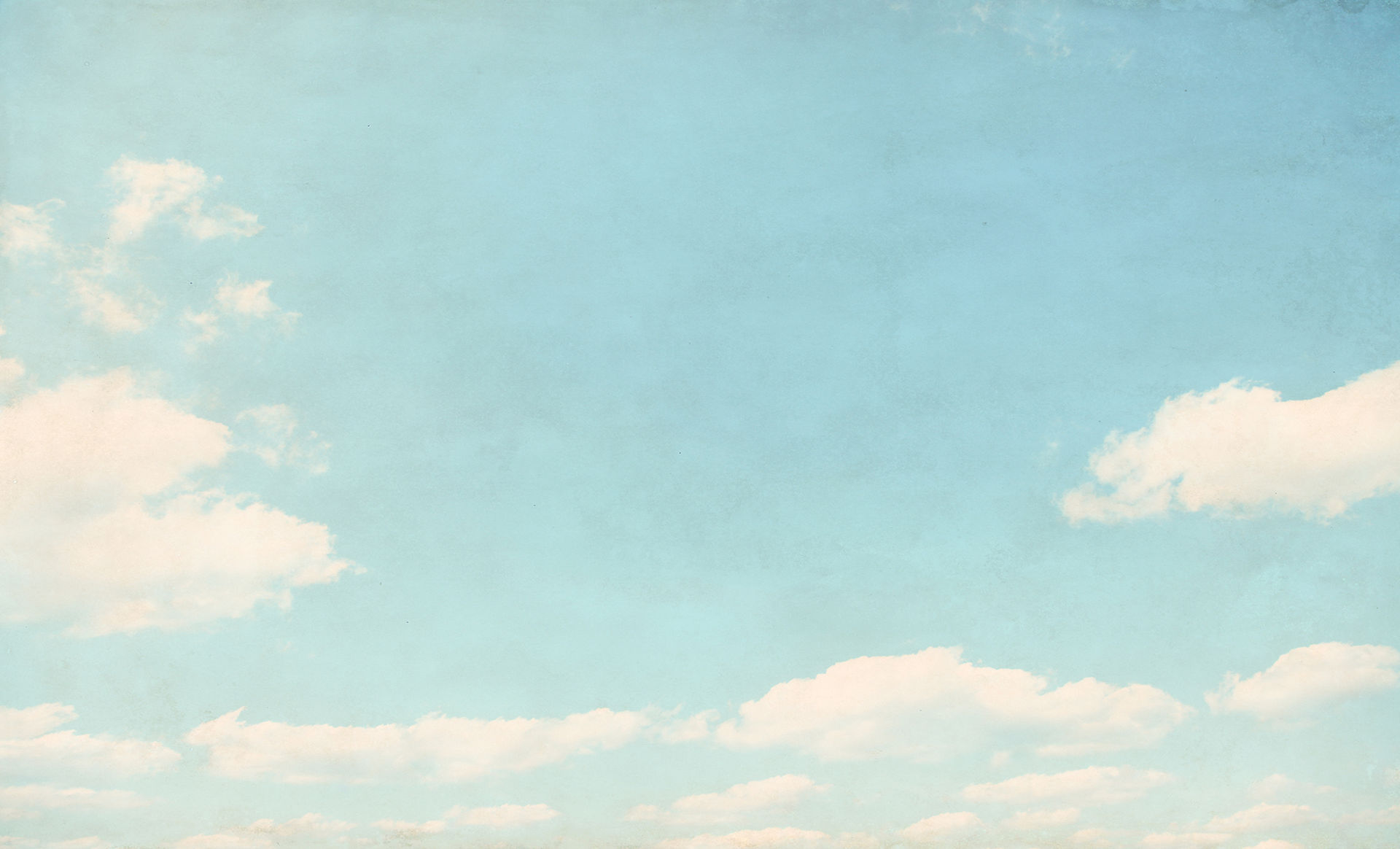
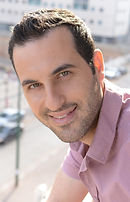
ALDROID
While steep growth is reported in the creation of new unknown malware aimed at compromising smartphones, these devices are very dependent on anti-virus solutions due to their resource limitations. Currently, the detection of new unknown malwares is a time-consuming and costly task for anti-virus vendors. Using manually crafted signatures, the anti-virus tool can only identify known malware instances and similar variants. However, to identify new unknown malware for updating the anti-virus signature repository, anti-virus vendors need to deal with vast quantities of new applications on a daily basis. Machine learning algorithms have been used to address this task, however they must be efficiently and daily updated as well. To improve detection and updatability, we introduce a new framework, "ALDROID" and present our active learning (AL) methods on which the framework is based: "Exploitation" and "Combination." These methods identify the most informative applications and rank them from the most informative to the least, by this prioritization, only the most informative applications are sent to the security experts who manually inspect them and determine their label as malicious or benign. By selecting only new informative applications (benign and especially malicious), we reduce the labeling efforts of the security experts, and enable a frequent and efficient process of updating and upgrading the framework’s detection model. Consequently the signature repository of the anti-virus tool is updated with new and representative malwares and thus its detection capabilities are enhanced as well as the security of the widely used smartphones. The framework and method were evaluated using 27,250 benign and malicious Android applications. The results indicate that both of our AL methods outperformed other solutions such as the existing AL method and a commonly used solution, the heuristic engine. In particular, Exploitation and Combination efficiently acquired the largest number (NOMA) and largest percentage (POMA) of new malwares in all the acquisition amounts, while still preserving the detection models’ detection capabilities with high TPR and low FPR rates. On the final day of the experiment, while acquiring applications at a rate of 245 per day, Exploitation and Combination acquired 207 new malware which is double the amount of new malwares acquired by the heuristic engine (NOMA = 103) and 6.5 times more malwares acquired by the existing AL method (NOMA = 32).
